How to Win More with Data and Simple Tips in Betting
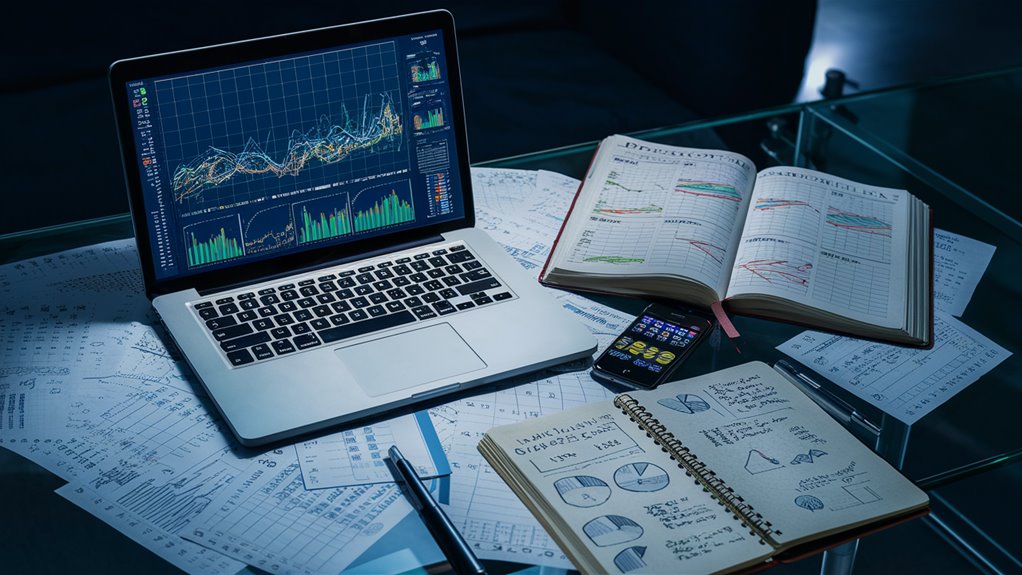
Use Easy Math for Smarter Bets
When you bet with data, you check many numbers from past games. You must collect lots of data and think about what it tells, like how teams have played before, and other big stuff that could shift the game.
Tools You Need for Betting with Stats
Get ahead by using smart data tools and connecting them to the web. Smart learning programs spot trends and gaps in the market that many miss. Look for key signs that can change your bet:
- Expected Value (EV)
- Closing Line Value (CLV)
- Past trend checks
- Team records
Build and Use Models
Learn stats coding with Python or R to create sharp betting tools. These help:
- Understand complex trends
- Handle data quickly
- Observe changes
- Take on deep math
Think of Outside Factors
To win in sports betting, know how things outside the game affect the outcome:
- Weather and its past effects
- How travel and tiredness affect teams
- Rest days between games
- Performance at home or away
Manage Your Money Wisely, Watch the Risk
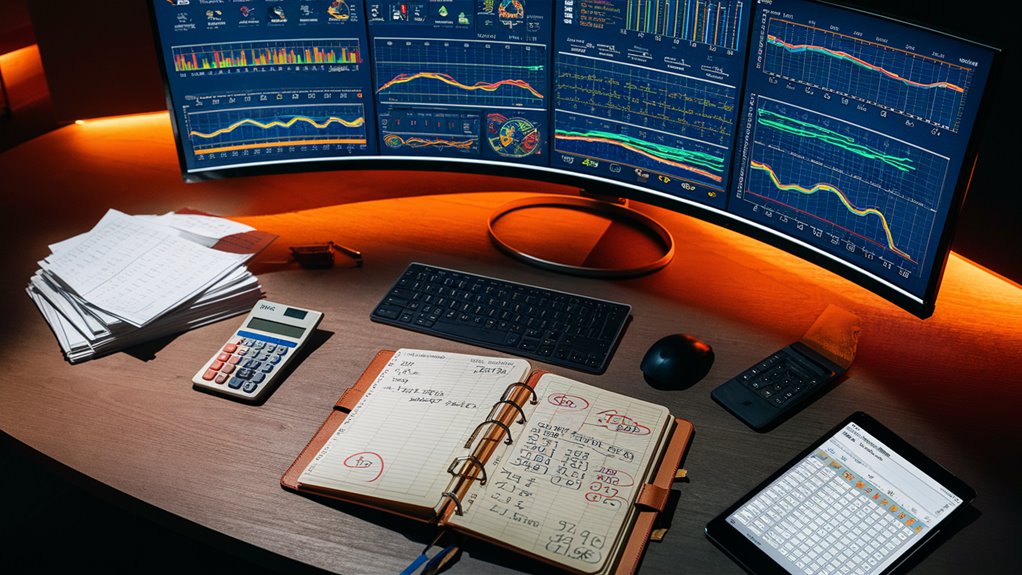
Be strict on how you manage your cash by:
- Using Kelly Criterion maths
- Changing your bet size based on risk
- Setting auto limits for losses
- Spreading your bets
Track your bets’ progress through detailed dashboards and adjust tactics based on solid numbers.
Main Betting Numbers to Know
The Important Stats for Betting
Top Betting Stats
Expected Value (EV) is a crucial number to track in betting.
This core math balances chance outcomes against bookie odds, by sports betting deliver multiplying chance to win by possible win money, then reducing by the product of chance to lose and what you first bet.
Market Stats
Closing Line Value (CLV) shows if you bet wisely.
It speaks to the gap between odds you got and final odds before the game, showing if you can predict well.
Return on Investment (ROI) and hold percent tell how well your money grows and if you can win over time.
Deep Number Analysis
Converting odds to percent shows good spots to bet.
Dive deep into past records, recent performances, and details like home/away data to craft sharp betting tools.
These tools identify good bet opportunities where the market’s odds don’t line up with real odds.